An Example: Applying A Life Table To A Problem
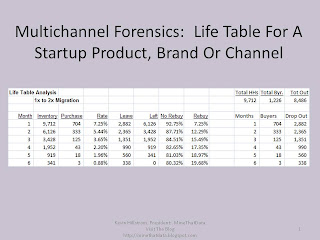
Expectations are a challenge, because we have to make an educated guess as to what might happen in the future.
Within a few months, we actually have enough data to make another educated guess ... we can predict the annual repurchase rate for a product, brand or channel.
In this case, our brand launched a new product. After six months, the product is not meeting expectations. Our CEO asks us to understand if the small number of customers who purchased this product are "loyal" to the product.
We ask our SAS programmers or information technology experts to run a query for us. We identify every customer who purchased our new product, to date. For each purchase, we bring along the customer_id, as well as the order date. The dataset is sorted by customer_id and order date.
Next, we re-shape the datasets, adding a column for the order date of the first purchase of merchandise from this product classification for a customer. We then scan the database. Any customer who ordered a second time has the order date for the second purchase put into another column.
If I purchased two times, my row of data looks something like this:
- Kevin .......... Date1 = 20080115 .......... Date2 = 20080507
- Kevin .......... Date1 = 20080115 .......... Date2 = NULL.
- Kevin Months = 04 .......... Second Purchase = YES
- Kevin Months = 04 .......... Second Purchase = NO
In the six months since launching the new product, just under twenty percent of customers buying the product chose to purchase the product again.
We can extend this relationship from six months through a year. Take a peek at the modeled relationship below:
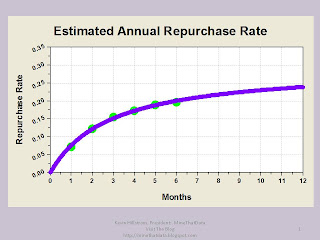
Notice the nice, smooth relationship exhibited by the data. The relationship indicates that, after twelve months, about 24% of customers will order the product again, putting the product squarely in "Acquisition Mode".
To answer the CEO's question ... it does not appear that customers are loyal to this product. It appears that if this product line is going to grow, it will grow by attracting new customers interested in a one-time purchase of this product line.
With as little as three months of purchase information, one can make a reasonably fair assessment of the anticipated annual repurchase rate of this product line.
And that, my friends, is one way that life tables can be applied to real world problems.
Labels: Acquisition Mode, Life Table, Multichannel Forensics